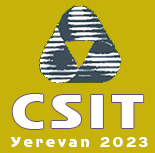
|
|  | |
|
Invited Speakers
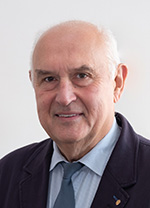 |
|
Gintautas Dzemyda
Prof. Dr. Habil.
Academician of the Lithuanian Academy of Sciences
Head of Division of Technical Sciences of the Lithuanian Academy of Sciences
Director of the Institute of Data Science and Digital Technologies of Vilnius University
Head of Cognitive Computing Research Group
|
Topic of the talk:
Visualization of Multidimensional Data: Methods, Software and Applications in Economy
Abstract:
Visualization is a part of data science, and serves as a mean for the sophisticated analysis of data. It ensures the human participation in decisions. Its application can uncover non-trivial knowledge from real-world data. We observe some regular structures and patterns in spending behavior for further fraudulent transactions detection. The methods and software for visualization of multidimensional data are reviewed. Particular attention is paid to nonlinear projection methods (MDS, Geometric MDS) and SOM-type artificial neural network. The emphasis is put on the web-based Orange solution that does not require computer programming skills. Analysis of Regional economic development and of Fraudulent transactions was performed using data visualization. These are the real problems where the graphical representations of multidimensional data find application and where the human participation is useful in decisions.
More information
|
|
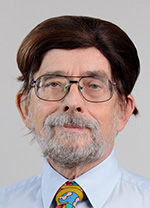 |
|
Gyula Katona
Prof. Doctor of the Mathematical Sciences
Academician of the Hungarian Academy of Sciences
Research professor at Alfred Renyi Institute of Mathematics, Hungarian Academy of Sciences
|
Topic of the talk:
On the identification of nearly monotone Boolean functions
Abstract:
The Boolean function f (x1,...,xn) of n variables maps the 0,1
vectors (x1,..., xn) : xi ∈ {0, 1}, i = 1,...,n into the set {0, 1}. We
write x = (x1,...,xn) ≤ y = (y1,...,yn) if and only if the inequality
holds componentwise. The Boolean function is called monotone if
x ≤ y implies f (x) ≤ f (y). A typical problem is to identify (that
is fully determine) the Boolean function f asking the values f (x) for
certain x’s. If nothing is known about the Boolean function then
f (x) obviously should be asked for each x. That is 2n questions are
necessary. This number can be reduced if it is known that the function
is monotone. Korobkov (1965) showed that in the worst case we need
at least ( n / [n/2] ) + ( n / [n/2+1] ) questions. Hansel (1966) proved that this
number of questions is really sufficient. We can say that the class of
monotone Boolean functions has complexity ( n / [n/2] ) + ( n / [n/2+1] ). The main
goal of the present work is to study the complexity of larger classes
of Boolean functions. E.g. we say that f is 2-monotone if x ≤ y does
not necessarily imply f (x) ≤ f (y), only when y − x has at least two
non-zero coordinates. We give estimates on the complexity of the class
of 2-monotone Boolean functions. Some more general cases are also
considered.
More information
|
|
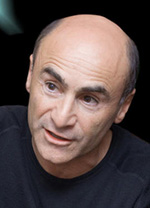 |
|
Ruben Abagyan
Prof. Doctor of the Biochemistry and Mathematics
Professor at the Skaggs School of Pharmacy and Pharmaceutical Sciences of University of California, and San Diego Supercomputer Center in San Diego
|
Topic of the talk:
High performance computing, 3D docking, AI and GPU pipelines to address challenges in the biotech and pharmaceutical industry
Abstract:
Here we review the next generation challenges of molecular docking and machine learning methods to enable efficient searchers of extremely large chemical catalogs, offered, synthesizeable, or ad-hoc generated. The challeges related with exponencially growing data sizes, the emergence of AI- and GPU- powered tools creates an opportunity to dramatically accelerate the new drug discovery process. Fast docking screens combined with multi-billion-compound chemical catalogs, and thousands of prepared docking/machine-learning models for individual targets, anti-targets, and important pharmacological properties facilitate rapid identification reasonable drug-like candidates with multi-target profiles for a diverse set of projects. Development and accelerating the internal coordinate mechanics (ICM) based flexible docking to 100 dockings per second, collaboration with Dr Max Totrov, Molsoft, was key to the success of the in silico stage of the lead discovery pipeline. Examples of successful application of the pipeline followed by rigorous experimental validation, include a new cancer target related to NALCN channel complex in collaboration with Dr. Jason Sicklick’s lab at UCSD, kinases associated with Tau in Alzheimer’s disease in collaboration with Dr. Andrea Cavalli’s lab at IIT and EPFL, and viral and parasitic proteases in collaboration with Drs. O’Donohue and A. Chavez labs at UCSD.
More information
|
| |
|